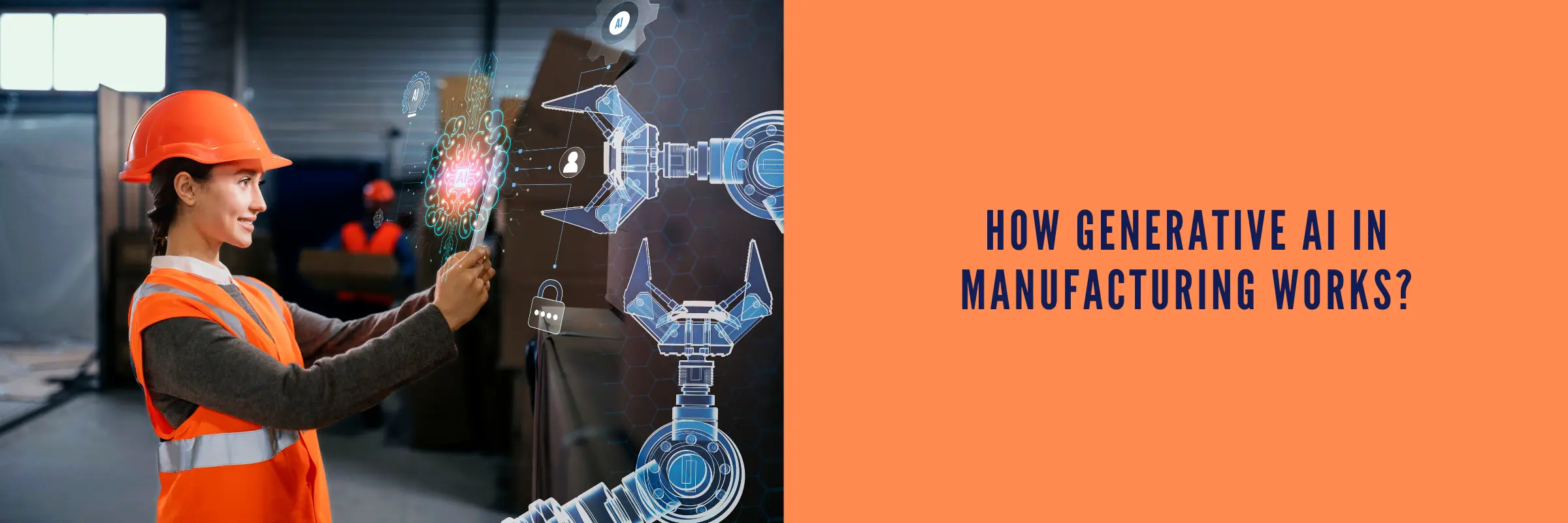
How Generative AI in Manufacturing Works: Use Cases, Examples
Manu Jain | February 28, 2024 , 10 min read
Table Of Content
Imagine a world where data isn’t just about numbers and figures. Instead, it shapes the very core of your operations. That’s precisely what generative AI in manufacturing brings to the table.
Still not clear?
This subset of AI goes beyond crunching numbers. It interprets data to help you make smarter decisions at every step of the product lifecycle, from design and engineering to manufacturing and beyond.
For instance, manufacturing giant General Motors is already taking the generative AI leap. They use tailored algorithms to optimize vehicle parts and reduce their weight.
How?
By generating different designs and putting them through virtual real-world tests.
The result?
Super-efficient, lighter, and tougher vehicle parts that are also cost-effective.
Given its transformative abilities, it’s no surprise that the global generative AI in manufacturing market size is expected to reach around $6.3 million by 2032.
However integrating generative AI into your manufacturing processes can be challenging, especially in domains where communication and emotional understanding are crucial.
In this blog, we’ll explain everything you need to know to leverage this transformative technology for your business advantage.
What is Generative AI in Manufacturing?
- What is Generative AI in Manufacturing?
- Benefits of Generative AI in Manufacturing
- How is Generative AI used in the Manufacturing Industry?
- Challenges of Generative AI in Manufacturing?
- Case Study: Generative AI in Manufacturing Examples
- How Can ScaleupAlly Help?
- Frequently Asked Questions (FAQs)
- Similar Articles Around Generative AI
Generative AI in manufacturing involves using artificial intelligence techniques, such as generative adversarial networks (GANs) or other generative models, to create or generate new designs, patterns, or solutions in the manufacturing process.
This technology allows manufacturers to produce innovative product designs while adhering to specific constraints or input criteria.
Generative AI can explore a wide range of possibilities to generate new solutions. It can also optimize manufacturing processes by generating efficient production schedules, minimizing waste, and enhancing quality control measures.
Furthermore, it can assist in selecting the most suitable materials for a particular product or application by considering factors such as strength, durability, and cost.
Benefits of Generative AI in Manufacturing
Generating AI offers numerous advantages in the manufacturing industry. Here are a few significant ones:
1. Product Design and Innovation
Generative AI can assist in product design by generating innovative concepts and iterating designs based on specified criteria and constraints. Car manufacturers can use generative AI to design a new engine component. The AI generates multiple design options, considering factors like performance, cost, and manufacturability, helping the manufacturer create a more efficient and cost-effective component.
2. Optimized Manufacturing Processes
Generative AI can optimize manufacturing processes by analyzing production data and identifying areas for improvement. Consider a furniture manufacturer that uses generative AI to analyze production line data. The AI identifies bottlenecks in the process and suggests changes to improve efficiency, such as rearranging workstations or adjusting production schedules.
3. Supply Chain Optimization
Generative AI can optimize supply chains by analyzing demand forecasts, inventory levels, and transportation routes to reduce costs and improve efficiency. A consumer electronics manufacturer can use generative AI to optimize its supply chain. The AI analyzes demand patterns and transportation costs to recommend the most cost-effective inventory levels and transportation routes.
4. Quality Control
Generative AI can improve quality control by analyzing real-time production data to detect defects and anomalies. Example: A food processing plant uses generative AI to monitor its production line. The AI analyzes images of food products to detect defects, such as bruises or discolouration, ensuring that only high-quality products are shipped to customers.
Curious about Generative AI Costs?
Our CTO decodes the top 10 FAQs in a free, easy-to-read PDF.
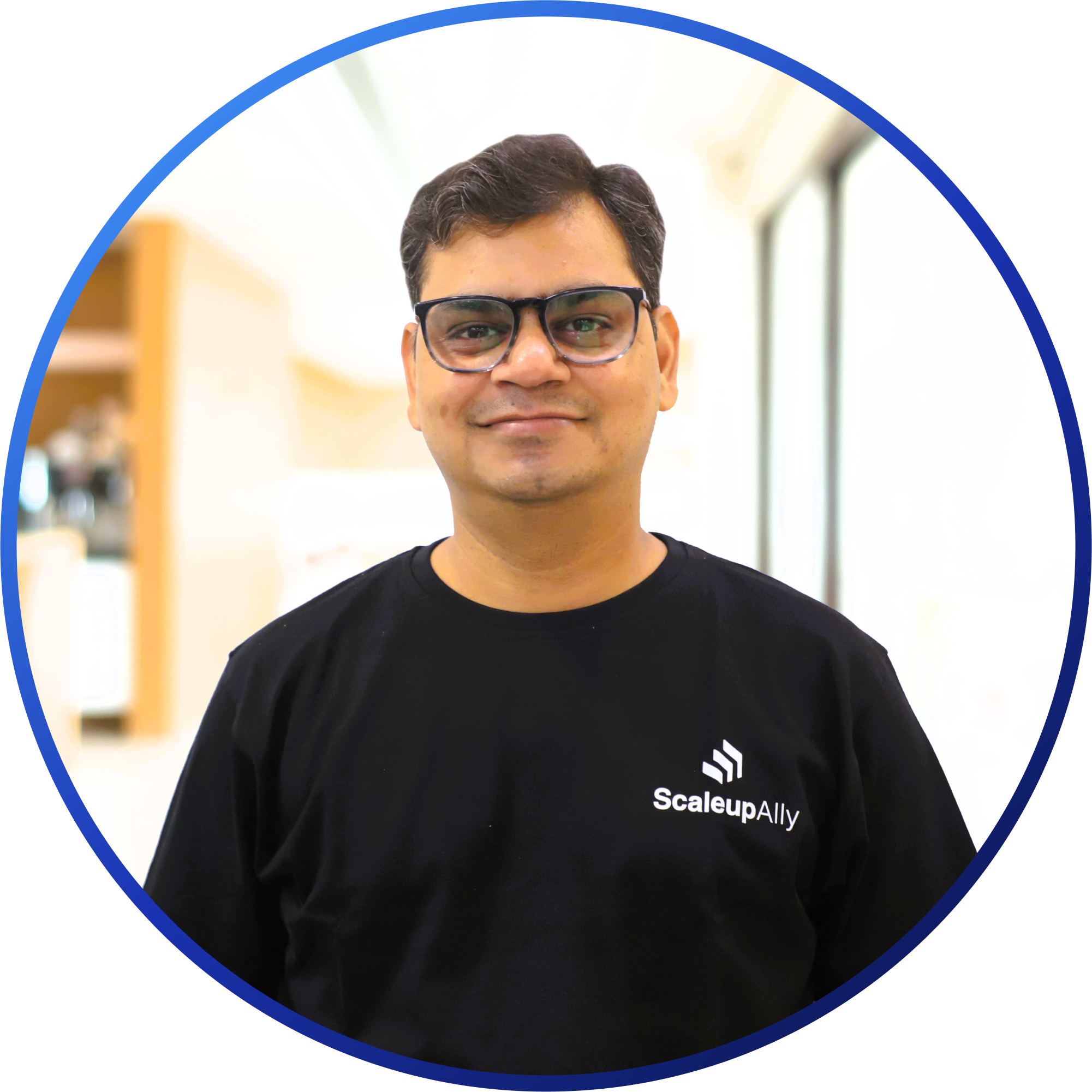
How is Generative AI used in the Manufacturing Industry?
Generative AI is used in the manufacturing industry for different purposes. Some of its common applications include:
1. Product Design
Generative AI can create new product designs based on specified parameters, optimizing for performance, cost, and manufacturability. For example, Airbus used generative design software to create a concept for a partition that separates the passenger cabin from the galley, reducing weight and improving efficiency.
2. Process Optimization
AI can optimize manufacturing processes by analyzing data from sensors and other sources to identify inefficiencies and suggest improvements. BMW uses AI to optimize the cooling of its electric vehicle batteries during manufacturing, improving energy efficiency and reducing costs.
3. Quality Control
r eGenerative AI can be used for quality control by analyzing images or sensor data to detect product defects. For example, Siemens uses AI to inspect wind turbine blades for defects, improving the quality of the blades and reducing the need for manual inspection.
4. Supply Chain Management
AI can optimize supply chains by analyzing data on demand, inventory levels, and transportation routes to minimize costs and maximize efficiency. IBM has used this to their advantage. They use AI to optimize its supply chain for semiconductor manufacturing, reducing costs and improving delivery times.
5. Predictive Maintenance
AI can be used for predictive maintenance by analyzing sensor data to predict when equipment is likely to fail, allowing for proactive maintenance. For example, GE uses AI to predict when its gas turbines need maintenance, reducing downtime and maintenance costs.
6. Customization
Adidas uses AI to create customized running shoes for customers, improving customer satisfaction and brand loyalty. Generative AI can enable mass customization by creating unique designs for individual customers based on their preferences.
Challenges of Generative AI in Manufacturing?
Generative AI has numerous advantages in the manufacturing industry. However, it also poses various challenges such as:
1. Data Quality and Quantity
Generative AI models require high-quality data to generate accurate and useful designs or solutions. Ensuring the availability and quality of such data can be challenging, especially for complex manufacturing processes.
2. Computational Resources
Training generative AI models require significant computational resources, including powerful hardware and specialized software. This can be costly and may require expertise to manage effectively.
3. Interpretability
Generative AI models can produce complex designs or solutions that are difficult to interpret or understand. This can make it challenging for manufacturers to trust the output of these models and incorporate them into their processes.
4. Ethical Considerations
As with any AI technology, generative AI raises ethical concerns, such as bias in training data or potential misuse. Manufacturers must carefully consider these ethical implications when deploying generative AI in their operations.
5. Integration with Existing Systems
Integrating generative AI into existing manufacturing systems can be complex, requiring compatibility with legacy systems and processes. This can pose challenges in terms of implementation and scalability.
6. Security
Generative AI models, like all AI systems, can be vulnerable to attacks and manipulation. Manufacturers must ensure the security of their generative AI systems to protect against potential threats.
7. Regulatory Compliance
The use of generative AI in manufacturing may be subject to regulatory requirements, such as data privacy and product safety standards. Manufacturers must ensure compliance with these regulations when deploying generative AI systems.
Case Study: Generative AI in Manufacturing Examples
Here are some real-life instances of manufacturing industries using generative AI:
1. Autodesk and General Motors (GM)
Autodesk, a software company, collaborated with General Motors to use generative design software to develop a new seatbelt bracket for the Chevrolet Venture. The software generated dozens of design options based on input criteria such as weight, strength, and cost. This approach resulted in a bracket design that was 40% lighter and 20% stronger than the original design, demonstrating the potential of generative AI in optimizing product designs.
2. Volkswagen
Volkswagen utilized generative design software to optimize the design of a water pump wheel for its vehicles. The software generated thousands of design options, which were then analyzed using computational fluid dynamics (CFD) simulations to identify the most efficient design. This approach resulted in a water pump wheel that was 20% lighter and 25% more efficient than the original design, demonstrating the potential of generative AI in improving product performance and efficiency.
How Can ScaleupAlly Help?
ScaleupAlly is an AI expert that can revolutionize your manufacturing business with Generative AI. Our team has years of experience creating customized solutions catering to your manufacturing needs.
What sets us apart is our focus on customization. We design AI solutions that integrate seamlessly into your existing processes, increasing efficiency and productivity. Our flexible approach ensures our solutions can grow and evolve with your business.
Our commitment to cost-effective manufacturing software development means you can leverage AI without exceeding your budget.
Frequently Asked Questions (FAQs)
Q: How is generative AI different from traditional design methods?
Generative AI differs from traditional design methods in that it can explore a much larger design space and generate innovative solutions that may not be apparent through manual design. It can also simultaneously optimize designs based on multiple criteria, leading to more efficient and effective designs.
Q: How does generative AI enable predictive maintenance in manufacturing?
Generative AI enables predictive maintenance in manufacturing by analyzing data from sensors to predict when equipment is likely to fail. This allows manufacturers to perform proactive maintenance, reducing downtime and maintenance costs.
Q: How can manufacturers get started with implementing generative AI in their operations?
Manufacturers can start implementing generative AI by identifying areas in their operations where AI can provide the most value, such as product design optimization or process improvement. They can then start with small pilot projects to test the technology and gradually scale up as they gain more experience and confidence in its capabilities.
Similar Articles Around Generative AI
Related Blogs
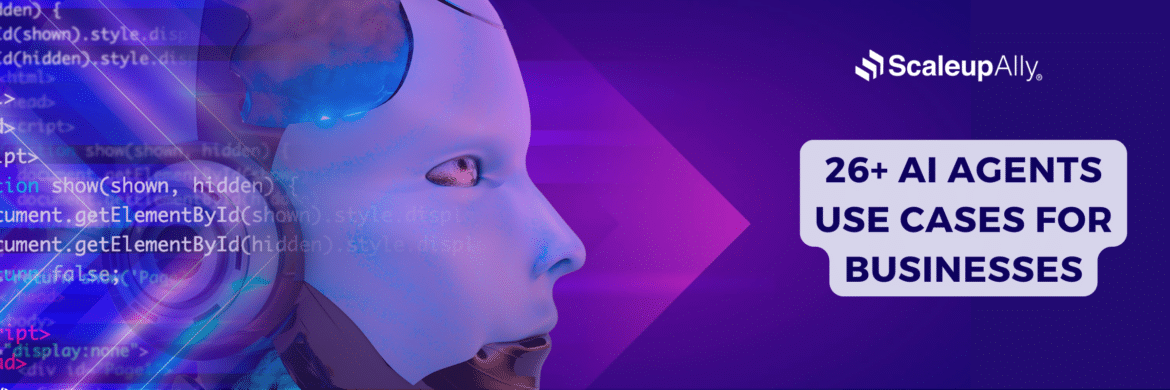
26+ AI Agents Use Cases for Businesses
Explore the best AI agent use cases for businesses, along with key benefits, challenges and best practices to optimize operations and drive business growth.
Manu Jain
Apr 23 ,
16 min read
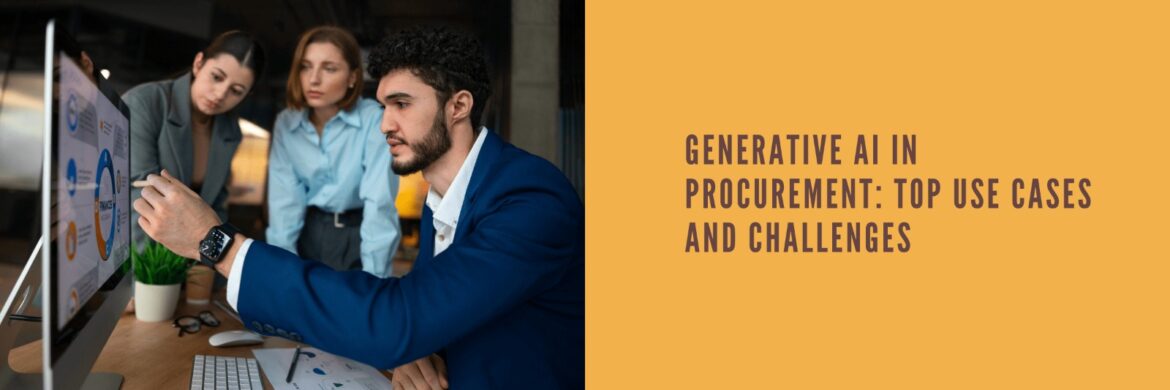
Generative AI in Procurement: Top Use Cases and Challenges
Generative AI in Procurement: In this article, Discover benefits, use cases for cost savings, challenges for risk reduction and the future it has in procurement.
Manu Jain
Apr 3 ,
14 min read
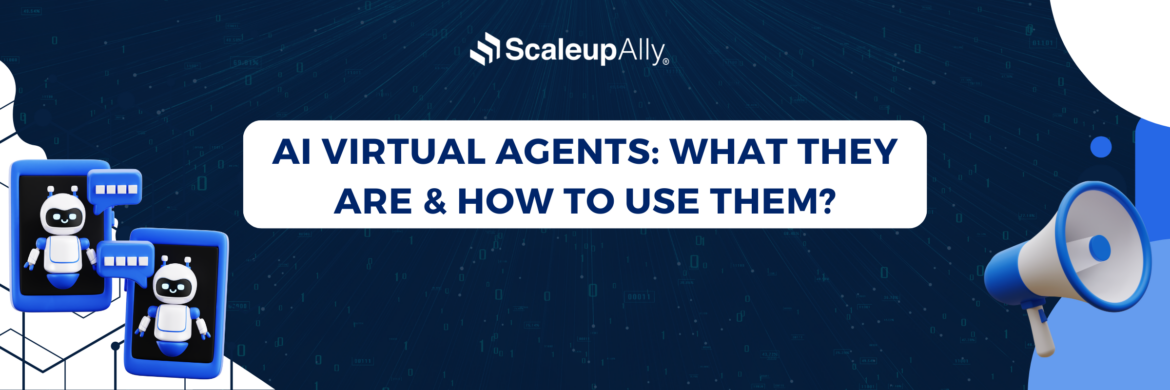
AI Virtual Agents: What They Are & How To Use Them?
Learn how AI virtual agents work, their features, key differences from chatbots, business benefits, and top use cases that can improve automation.
Manu Jain
Mar 3 ,
13 min read